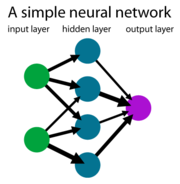
http://upload.wikimedia.org/wikipedia/en/thumb/1/1d/Neural_network_example.png/180px-Neural_network_example.png
A code was already prepared by Jeric Tugaff and was only modified for this activity's purpose. The values used are as 4 input feature vectors (normalized between 0-1) both from 4 training objects for each class and 4 test objects for each class.
The neural network is trained using the training set. The code is expected to output values close to
[0 0 0 0 1 1 1 1]
meaning the first four test object will be classified as belonging to the pillows class and the second set of test objects will be classified under the kwek-kwek class. The output is as follows. From this table, 100% classification of the test objects is obviously obtained.
//code
chdir('C:\Documents and Settings\VIP\Desktop\ap186\A20');
training = fscanfMat('training.txt');
test = fscanfMat('test.txt');
//training
mntr = min(training, 'c');
tr2 = training - mtlb_repmat(mntr, 1, 8);
mxtr = max(tr2, 'c');
tr2 = tr2./mtlb_repmat(mxtr, 1, 8);
//test
mnts = min(test, 'c');
ts2 = test - mtlb_repmat(mnts, 1, 8);
mxts = max(ts2, 'c');
ts2 = ts2./mtlb_repmat(mxts, 1, 8);
tr_out = [0 0 0 0 1 1 1 1];
N = [4, 10, 1];
lp = [0.1, 0];
W = ann_FF_init(N);
T = 400;
W = ann_FF_Std_online(tr2,tr_out,N,W,lp,T);
//x is the training t is the output W is the initialized weights,
//N is the NN architecture, lp is the learning rate and T is the number of iterations
// full run
ann_FF_run(ts2,N,W) // classification output
//end code
---
Thanks Jeric Tugaff for helping me understand how neural network works and for helping me with the program.
---
Rating 7/10 since I implemented the program correctly but was very dependent on Jeric's tutorial and discussion. :)
chdir('C:\Documents and Settings\VIP\Desktop\ap186\A20');
training = fscanfMat('training.txt');
test = fscanfMat('test.txt');
//training
mntr = min(training, 'c');
tr2 = training - mtlb_repmat(mntr, 1, 8);
mxtr = max(tr2, 'c');
tr2 = tr2./mtlb_repmat(mxtr, 1, 8);
//test
mnts = min(test, 'c');
ts2 = test - mtlb_repmat(mnts, 1, 8);
mxts = max(ts2, 'c');
ts2 = ts2./mtlb_repmat(mxts, 1, 8);
tr_out = [0 0 0 0 1 1 1 1];
N = [4, 10, 1];
lp = [0.1, 0];
W = ann_FF_init(N);
T = 400;
W = ann_FF_Std_online(tr2,tr_out,N,W,lp,T);
//x is the training t is the output W is the initialized weights,
//N is the NN architecture, lp is the learning rate and T is the number of iterations
// full run
ann_FF_run(ts2,N,W) // classification output
//end code
---
Thanks Jeric Tugaff for helping me understand how neural network works and for helping me with the program.
---
Rating 7/10 since I implemented the program correctly but was very dependent on Jeric's tutorial and discussion. :)